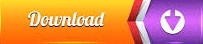
In areas such as medical imaging, the availability of pre-trained models are almost negligible. ML models are good as the data it is fed with. Equipping machine learning algorithms with human-like reasoning is tricky. Most of the AI R&D endeavours converge at one thing - making AGI possible. Though responsible and explainable AI practices overlap in many ways the end goal is to enable transparency in AI-based decision making. Whereas, explainable AI featured on the descending curve of inflated expectations. Responsible AI too, like Generative AI featured in the first segment bordering on ‘peak of inflated expectations’. To make the process of inculcating responsible AI systems more viable, tools such as Model cards, AI 360 and many others have been released by companies like Google, IBM etc. Be it medical diagnosis or credit card risk estimation, the amount of personal information that is processed can be very sensitive, and this is being addressed in the organisations which are serious about the implementation of explainability.įrom inductive biases to GDPR compliance, AI enterprises have many things to take care. Apart from the debugging and auditing of the models, data scientists need to look out for meeting data privacy standards in the context of explainability. With increasing business applications such as autonomous driving and medical diagnosis, there is a growing demand from the stakeholders to know what is at stake. Machine learning algorithms are infamous for their black-box nature.

Stage: Innovation Trigger & Peak of inflated expectations resp.

For good or for worse, these networks are here to stay for a while now. Generative networks are all fun and games until they start interfering with matters of national interest. Malicious online players can now generate disinformation in the form of images and videos that can fool many. The proliferation of generative networks has had many unwanted results. In the Gartner Hype Cycle, Generative AI featured in the ‘innovation trigger’ segment of the graph. These networks are considered to be one of the critical turning points in the history of ML. All this thanks to Generative Adversarial Networks (GANs). 2| Generative AIĪI can now paint auction-worthy art, generate songs and even create faces of people who never existed. The safe, secure and real-time output is the theme of the modern world. Whereas, Google’s Coral toolkit can be leveraged to bring machine learning to edge.
#GARTNER HYPE CYCLE ARTIFICIAL INTELLIGENCE 2019 PORTABLE#
As we approach an era of IoT, 5G and portable medical devices, it is crucial to facilitate developers to develop and deploy edge applications quickly.įor example, with the NVIDIA Jetson AGX Xavier developer kit, as shown above, one can easily create and deploy end-to-end AI robotics applications for manufacturing, retail, smart cities, and more. Thanks to the efforts of top companies to place supercomputers in the pockets, Edge computing systems have garnered significant attention. In this article, we will focus exclusively on the AI segment of this survey. “This Hype Cycle highlights technologies that will significantly affect business, society and people over the next five to 10 years,” said Brian Burke, Research VP, Gartner.įrom algorithmic trust to advanced AI, the Gartner’s hype cycle features many new technologies.

It includes technologies that can completely change the direction of human civilization.

Last week, Gartner released a unique hype cycle for emerging technologies.
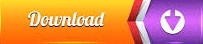